Unlocking Business Potential Through Data Annotation
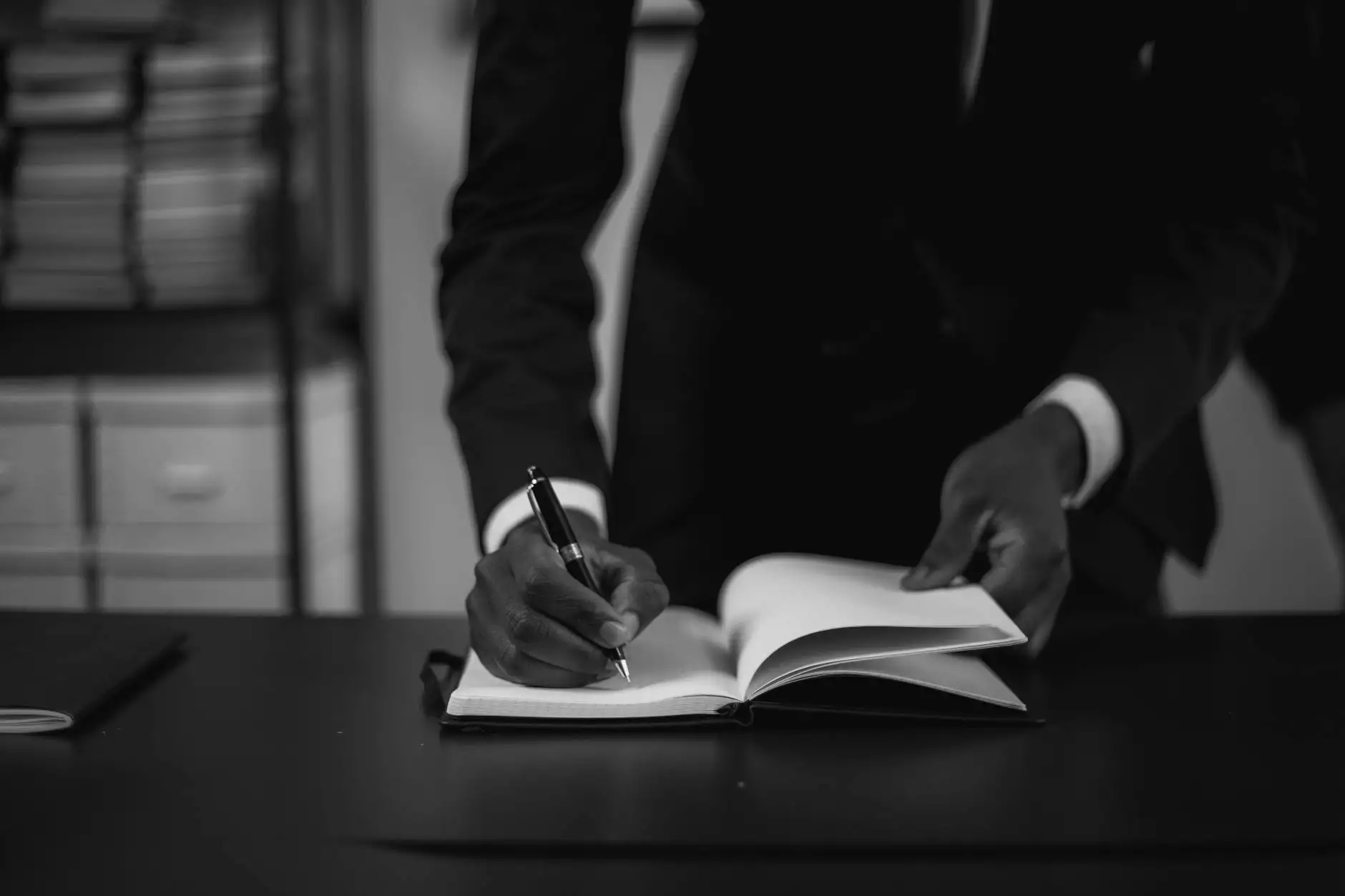
Data annotation is revolutionizing the way businesses operate. It’s an essential process that enhances the performance of machine learning models, ultimately leading to better decision-making and more efficient operations. As you dive deeper into this topic, you will discover why data annotation is not just a technical requirement but a strategic advantage for businesses across various industries.
Understanding Data Annotation
At its core, data annotation refers to the process of labeling or tagging data to make it machine-readable. This process is crucial for training machine learning models, allowing computers to interpret and understand the data they process. By providing context and meaning to raw data, businesses can significantly improve how they utilize technologies such as artificial intelligence (AI) and machine learning (ML).
The Importance of Data Annotation in Business
In today’s digital age, the volume of data generated is unprecedented. According to recent studies, data annotation plays a pivotal role in transforming this raw data into valuable insights. Here are some key reasons why data annotation is vital for your business:
- Enhanced Machine Learning Accuracy: Properly annotated datasets improve the accuracy of machine learning algorithms, leading to better predictions and decisions.
- Improved Customer Insights: Analyzing annotated data enables businesses to understand customer behavior, preferences, and trends more effectively.
- Efficiency in Operations: Automated systems equipped with well-annotated data can streamline processes, reducing the time and cost involved in manual analysis.
- Competitive Edge: Businesses that leverage data annotation can gain a significant advantage by making informed decisions faster than their competitors.
Types of Data Annotation Techniques
Various techniques can be employed in data annotation, each tailored to meet specific needs. Here are some prevalent types:
1. Image Annotation
Image annotation involves labeling images with tags or descriptions, making it crucial for computer vision tasks. This technique is commonly used in applications such as:
- Object Detection: Identifying and classifying objects within an image.
- Semantic Segmentation: Dividing an image into segments and associating them with a class label.
- Facial Recognition: Annotating images of faces for identity verification systems.
2. Text Annotation
Text annotation is vital for natural language processing (NLP) applications. It involves labeling text data, making it easier for algorithms to understand linguistic structures. Key uses include:
- Sentiment Analysis: Determining the sentiment behind a piece of text.
- Entity Recognition: Identifying named entities within a body of text.
- Topic Classification: Assigning categories based on the content of the text.
3. Video Annotation
Video annotation is increasingly important with the rise of video content. It allows machines to understand video sequences, enhancing applications such as:
- Activity Recognition: Identifying specific actions within video clips.
- Object Tracking: Following objects across frames in a video.
- Scene Segmentation: Dividing videos into coherent segments.
Implementing a Successful Data Annotation Strategy
To leverage data annotation effectively, businesses need a solid strategy. Here are steps to consider:
1. Define Objectives
Before embarking on a data annotation project, it’s essential to define clear objectives. Ask yourself:
- What do I hope to accomplish with this annotated data?
- What specific models or algorithms will utilize this data?
- What insights do I wish to derive from the analysis?
2. Choose the Right Tools
Selecting the proper tools is crucial for effective data annotation. Here are some preferred tools available:
- KeyLabs.ai: A robust platform providing automated solutions for data annotation across various media types, ensuring accuracy and efficiency.
- Labelbox: A versatile tool that offers a collaborative environment for data annotation, suitable for teams across the globe.
- SuperAnnotate: A tool with advanced features for annotating images, videos, and texts, focusing on enhancing machine learning workflows.
3. Train Annotations Teams
While automated tools can significantly aid in data annotation, human oversight is irreplaceable, particularly for nuanced tasks. Training your annotation team to understand specific requirements is vital. Ensure your team is familiar with:
- Industry standards for data labeling.
- Best practices in annotation techniques relevant to your business.
- The technology and tools they will be using on a daily basis.
Challenges in Data Annotation
While data annotation provides immense benefits, it also presents challenges that businesses must navigate:
1. Quality Control
Ensuring the quality of annotated data is a significant challenge. Human errors can lead to inconsistencies that negatively impact model performance. Regular review processes and the use of quality assurance metrics can help mitigate this risk.
2. Scalability
As the volume of data grows, so does the need for scalable annotation solutions. Businesses must invest in tools and technologies that can handle large datasets efficiently without compromising quality.
3. Keeping Up with Technology
The landscape of machine learning and data annotation is constantly evolving. Businesses must stay updated on the latest technologies and methods to ensure they remain competitive and effective in their data strategies.
The Future of Data Annotation
As AI and machine learning technologies advance, the role of data annotation will become even more critical. Here are a few trends to watch:
1. Increased Automation
Automated annotation tools will continue to evolve, allowing for faster and more accurate data processing. This evolution will enable businesses to focus more on strategic decision-making rather than manual tasks.
2. Crowdsourcing
Crowdsourcing for annotation tasks is anticipated to grow, allowing businesses to leverage a global workforce to achieve scale and diversity in annotations. This shift will likely lead to innovative approaches to tackling the complexity of data annotation.
3. Greater Emphasis on Ethics and Privacy
As the importance of data privacy grows, businesses will need to adopt ethical practices in data annotation, ensuring that personal data is treated with utmost care. Transparency in how data is annotated and used will become a priority.
Conclusion
In conclusion, data annotation is an indispensable tool for businesses striving to harness the power of AI and machine learning. By implementing robust annotation strategies, leveraging the right tools like KeyLabs.ai, and staying informed about industry trends, businesses can transform their data into actionable insights, paving the way for greater success in today’s competitive landscape. The journey of embracing data annotation is not merely about technology; it’s about embracing innovation to unlock potential.